Relighting And Color Grading With Machine Learning
Continuity can be a challenge for shoots that are plagued by varying weather conditions, where, for instance, the pick-up shots are in bright sunlight but the core footage was shot under an even layer of cloud.
The cheapest and perhaps most notorious post-fix for 'night-time' footage, familiar to any viewer of old movies, was altering the F-stop settings at processing time in order to over-expose the film and produce a dark and gloomy effect from material that was shot in broad daylight – known as 'day for night'[1].
Neural networks have been brought to bear on the problem for a few years now. In 2019 a Google-led academic collaboration presented a novel neural network that implemented a rudimentary process for relighting, though the results were not entirely convincing[2].
In 2020 another collaboration, this time between Amazon, Adobe and the University of Maryland, developed a relighting algorithm capable of working on portraits as large as 1024x1024 – which is pretty HD for the image synthesis space, at least at the moment.
Google is conducting other research[3] in this area, and has built a dedicated capture rig called the Google Light Stage, to aid the generation of datasets for machine learning architecture capable of translating facial subjects between differing lighting set-ups.
The technology is integrated[4] as a post-capture feature in Google Photos.
Research from 2020 out of the Max Planck Institute for Intelligent Systems, and in collaboration with EPIC games, suggests an end-to-end relighting architecture, trained on light-stage captures, that's capable of reproducing shadows with fidelity. The researchers contend that this work is the first to achieve realistic directional shadows from strong light sources.
It's early days for this particular pursuit - relighting a scene even to the point of including credible shadows is an ambitious challenge for machine learning, and most research centers around light stage capture rather than higher-concept computer vision approaches.
NVIDIA's Vid2Vid architecture is arguably capable of more convincing work, albeit at lower resolutions and on less challenging subjects than the human face and form.
The 2017 paper Unsupervised Image-to-Image Translation Networks, and the accompanying video (below), demonstrate the potential power of neural networks to radically relight footage, and even to change the season in which it was taken.
Colorlab.ai currently uses machine learning to power its grading workflows, training on datasets that seek to distill human visual models of perception, and claiming[5] to be able to develop an applicable grading model for a project in a fraction of the conventional time.
Colorlab's neural network can even import a reference image, analyze and train on it, and then apply its inferred style to footage.
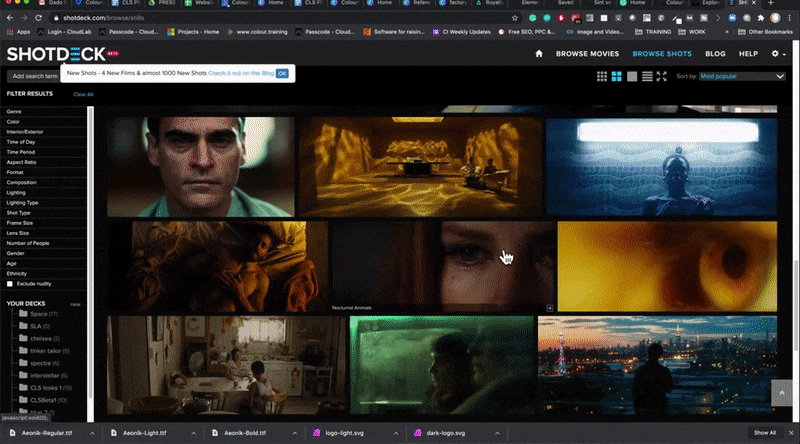
The company was founded by professional colorist Dado Valentic, who decided to pivot from a cloud-based approach and port the project to home-use after the advent of COVID-19.
Using OpenML and Metal 2, the end-user implementation is reported to actually run faster on a MacBook Pro than on professional grade custom hardware.
Colorlab offers support for Avid AAF, Tangent Panels, Arri RAW, Nobe Omniscope, and a growing number[6] of formats and import integrations for major packages.
Further reading:
Towards High Fidelity Face-Relighting with Realistic Shadows
https://github.com/andrewhou1/Shadow-Mask-Face-Relighting
[1] Cinematography Tip: Why ‘Day for Night’ Is a Horrible Idea – Caleb Ward, Premium Beat, 29th September 2015. https://www.premiumbeat.com/blog/cinematography-tip-why-day-for-night-is-a-horrible-idea/
[2] Single Image Portrait Relighting - SIGGRAPH 2019 – Yun-Ta Tsai, YouTube, 3rd May 2019.
Researchers Developed an AI that Can ‘Relight’ Portraits After the Fact – DL Cade, PetaPixel, 16th July 2019.
[3] Portrait Light: Enhancing Portrait Lighting with Machine Learning - Yun-Ta Tsai and Rohit Pandey, Google Blog, 11th December 2020. https://ai.googleblog.com/2020/12/portrait-light-enhancing-portrait.html
[4] A new, more helpful editor in Google Photos - Zachary Senzer, Google Blog, 30th September 2020.
[5] https://nofilmschool.com/ai-comes-filmmaking-colourlab
[6] https://colourlab.ai/colourlab-ai/#roadmap